By Haziqa Sajid,
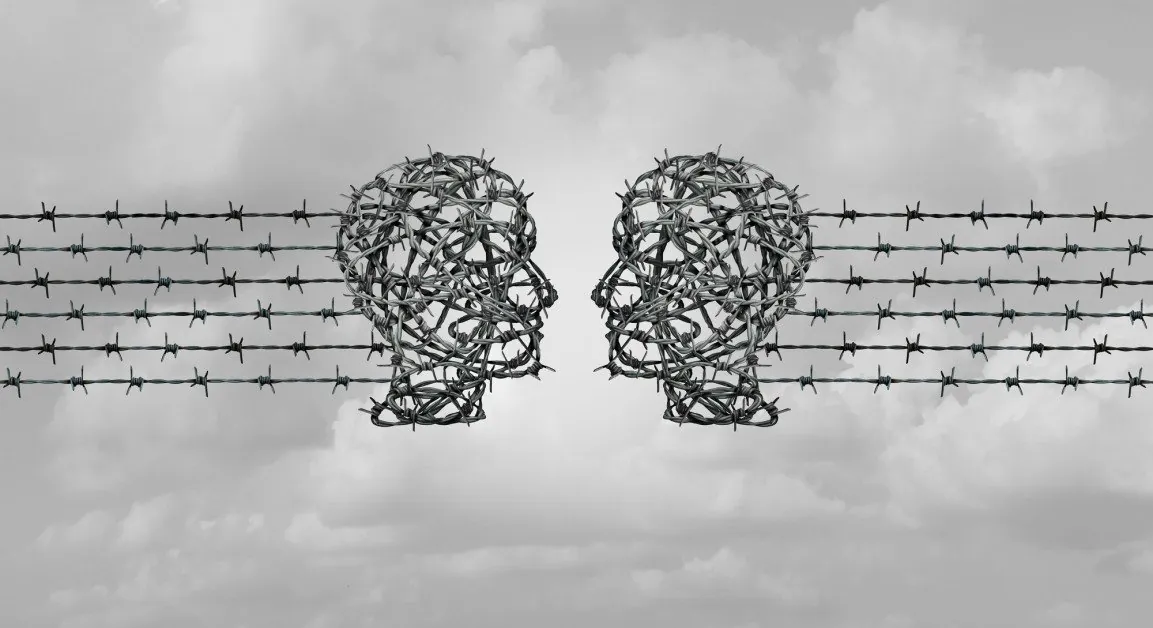
Today, the internet is the lifeblood of global communication and connection. However, with this unprecedented online connectivity, we also witness the dark side of human behavior, i.e., hate speech, stereotyping, and harmful content. These issues have permeated social media, online forums, and other virtual spaces, inflicting lasting damage on individuals and society. Hence, the need for hate speech detection.
According to the Pew Research Center, 41% of American adults say they have personally encountered internet abuse, and 25% are victims of severe harassment.To foster a more positive and respectful online environment, embracing proactive measures and leveraging the power of technology is imperative. In this regard, Artificial Intelligence (AI) provides innovative solutions to detect and tackle hate speech and stereotypes.
Limitations of Current Mitigation Techniques & The Need for Proactive MeasuresThe current measures to mitigate hate speech are limited. They cannot effectively curb the spread of harmful content online. These limitations include:
Reactive approaches, predominantly relying on human moderation and static algorithms, struggle to keep pace with the rapid dissemination of hate speech.
The sheer volume of online content overwhelms human moderators, resulting in delayed responses and missed instances of harmful rhetoric.
Also, contextual understanding and evolving language nuances pose challenges for automated systems to identify and interpret hate speech instances accurately.
To address these limitations and foster a safer online environment, a shift towards proactive measures is imperative. By adopting AI-powered measures, we can fortify our digital communities, encouraging inclusivity and a cohesive online world.
Identifying & Flagging Hate Speech Using AIIn the battle against hate speech, AI emerges as a formidable ally, with machine learning (ML) algorithms to identify and flag harmful content swiftly and accurately. By analyzing vast amounts of data, AI models can learn to recognize patterns and language nuances associated with hate speech, enabling them to categorize and respond to offensive content effectively.
To train AI models for accurate hate speech detection, supervised and unsupervised learning techniques are used. Supervised learning involves providing labeled examples of hate speech and non-harmful content to teach the model to distinguish between the two categories. In contrast, unsupervised and semi-supervised learning methods leverage unlabeled data to develop the model's understanding of hate speech.
Leveraging AI Counterspeech Techniques for Combatting Hate SpeechCounterspeech emerges as a powerful strategy to combat hate speech by directly challenging and addressing harmful narratives. It involves generating persuasive and informative content to promote empathy, understanding, and tolerance. It empowers individuals and communities to actively participate in creating a positive digital environment.
While specific details of individual counterspeech models may vary based on the AI technology and development approaches, some common features and techniques include:
Natural Language Generation (NLG): Counterspeech models use NLG to produce human-like responses in written or spoken form. The responses are coherent and contextually relevant to the specific instance of hate speech it is countering.
Sentiment Analysis: AI counterspeech models employ sentiment analysis to assess the emotional tone of the hate speech and tailor their responses accordingly. This ensures that the counterspeech is both impactful and empathetic.
Contextual Understanding: By analyzing the context surrounding hate speech, counterspeech models can generate responses addressing specific issues or misconceptions, contributing to more effective and focused counterspeech.
Data Diversity: To avoid biases and ensure fairness, counterspeech models are trained on diverse datasets representing various perspectives and cultural nuances. This helps in generating inclusive and culturally sensitive responses.
Learning from User Feedback: Counterspeech models can continuously improve by learning from user feedback. This feedback loop allows the model to refine its responses based on real-world interactions, enhancing its effectiveness over time.
Examples of Combating Hate Speech Using AIA real-world example of an AI counterspeech technique is the “Redirect Method” developed by Google's Jigsaw and Moonshot CVE. The Redirect Method uses targeted advertising to reach individuals susceptible to extremist ideologies and hate speech. This AI-powered approach aims to dissuade individuals from engaging with harmful content and promote empathy, understanding, and a shift away from extremist beliefs.
Researchers have also developed a novel AI model called BiCapsHate that acts as a potent tool against online hate speech, as reported in IEEE Transactions on Computational Social Systems. It supports a bidirectional analysis of language, enhancing context comprehension for accurate determination of hateful content. This advancement seeks to mitigate the damaging impact of hate speech on social media, offering the potential for safer online interactions.
Similarly, researchers at the University of Michigan have leveraged AI to combat online hate speech using an approach called Rule By Example (RBE). Using deep learning, this approach learns the rules of classifying hate speech from examples of hateful content. These rules are applied to input text to identify and predict online hate speech accurately.
Ethical Considerations for Hate Speech Detection ModelsTo maximize the effectiveness of AI-powered counterspeech models, ethical considerations are paramount. However, it is important to balance free speech and the prohibition of disseminating harmful content to avoid censorship.
Transparency in developing and deploying AI counterspeech models is essential to foster trust and accountability among users and stakeholders. Also, ensuring fairness is equally important, as biases in AI models can perpetuate discrimination and exclusion.
For instance, AI designed to identify hate speech can inadvertently amplify racial bias. Research found that leading hate speech AI models were 1.5 times more likely to flag tweets by African Americans as offensive. They are 2.2 times more likely to flag tweets as hate speech that are written in African American English. Similar evidence emerged from a study of 155,800 hate speech-related Twitter posts, highlighting the challenge of addressing racial bias in AI content moderation.
In another study, researchers tested four AI systems for hate speech detection and found all of them struggling to accurately identify toxic sentences. To diagnose the exact issues in these hate speech detection models, they created a taxonomy of 18 hate speech types, including slurs and threatening language. They also highlighted 11 scenarios that trip up AI, such as using profanity in non-hateful statements. As a result, the study produced HateCheck, an open-sourced data set of almost 4,000 examples, aiming to enhance the understanding of hate speech nuances for AI models.
Awareness & Digital LiteracyCombating hate speech and stereotyping demands a proactive and multidimensional approach. Hence, raising awareness and promoting digital literacy is vital in combatting hate speech and stereotypes.
Educating individuals about the impact of harmful content fosters a culture of empathy and responsible online behavior. Strategies that encourage critical thinking empower users to discern between legitimate discourse and hate speech, reducing the spread of harmful narratives. Also, equipping users with the skills to identify and effectively respond to hate speech is vital. It will empower them to challenge and counter harmful rhetoric, contributing to a safer and more respectful digital environment.
As AI technology evolves, the potential to address hate speech and stereotypes with greater precision and impact grows exponentially. Hence, it is important to solidify AI-powered counterspeech as a potent tool in fostering empathy and positive engagement online.
Source: Unite.AI
Original Content:
https://shorturl.at/hHRT5