By Martha Tsigkari
Martha Tsigkari is a senior partner and head of the Applied R+D group at Foster + Partners. She is also a Building the Future commissioner. In a special long read comment piece, she considers the significant challenges and huge possibilities that AI can bring to our industry
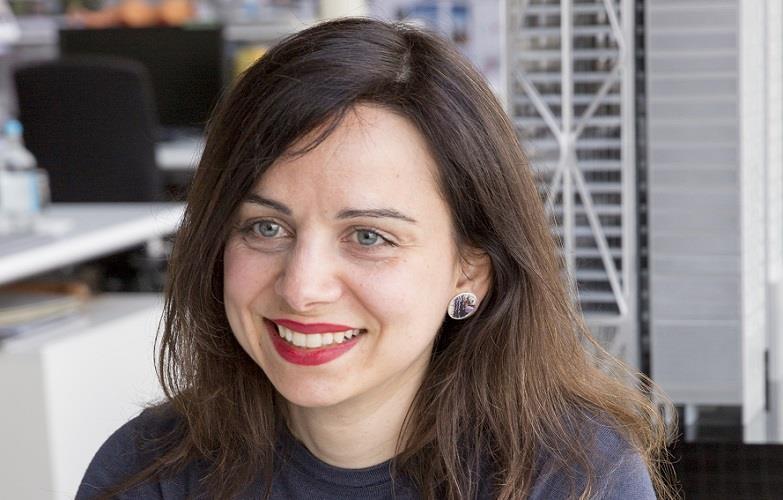
Disruptive technologies, of which artificial intelligence (AI) is currently a frontrunner, can be such charming underminers of our own certainty, irrevocably pushing us outside our comfort zone and forcing us to rethink all that we have been taking for granted. They are, in many ways, the white rabbit of our industry. And, like Alice in Wonderland, we can choose to ignore them. Or we can go down the rabbit hole.
Down the rabbit hole there is a great deal of uncertainty, unknowns and failure. But there are also a lot of possibilities. “I prefer the hell of chaos to the hell of order,” writes Wislawa Szymborska in her homonymous poem, and nothing is truer when it comes to disruption.
It is indeed within the creative chaos and outside of our comfort zones that we can reinvent paradigms. It is only by pushing our own boundaries that change is possible. And it is only by embracing change that we can bring new ideas to the table. But change can have unpredictable consequences. Think of the smartphone: a technology nobody knew that they needed and now not only everyone uses but it has also reshaped the way people interact by establishing the rise of social media.
AI is the superset of techniques powered by machine learning (ML) that enable machines to mimic human behavior So, what will the consequences of disruptive technologies, like AI, be in the Architecture Engineering Construction and Operation (AECO) industry? Before we discuss the particulars of AI in our industry, let us first place it in a wider framework.
In broad terms, AI is the superset of techniques powered by machine learning (ML) that enable machines to mimic human behaviour. You may have also heard of artificial general intelligence (AGI), or God-like AI, a computer system capable of generating new scientific knowledge and performing tasks as a human would.
The major, or rather more publicised, dramatis personae in the race towards AGI are DeepMind and OpenAI. DeepMind was acquired by Google for over half a million dollars in 2014. Since then, it has beaten the Go world champion and solved one of biology’s greatest unsolved problems. OpenAI, on the other hand, started as a non-profit competitor of DeepMind, before pivoting to be for-profit after Microsoft’s $1bn investment in 2019.
Of course, there are also other rising stars in the game, such as Anthropic, Cohere and Stability AI, who are all invested in developing closed or open-sourced Large Language Models (LLMs): natural language processing (NLP) programs trained through neural networks to perform various text completion tasks, having been given a well-crafted prompt.
The number of players in the field is steadily growing as the allure of these systems increases exponentially along with the promise of the positive change they will bring. However, it is important to note that the biggest player in this game is us. It is the data that we produce, the assets we create and the actions we take that train these systems.
Every single action in our life is underscored by the production of data that is being mined and used in a variety of waysData has become the currency of modern society. It is, in many ways, the most abundantly generated product of the 21st century. Every single action in our life is underscored by the production of data that is being mined and used in a variety of ways.
From identifying spam emails and suggesting what song to listen to, to defining our banking credit profile and making shopping recommendations, data and AI are guiding decision-making everywhere. Thousands of companies are leveraging the power of data through machine learning.
Aside from data, there are two more things that drive the rise of AI: the rate of the adoption of the technology and the rise in computational power.
The rate of AI adoption is incredible. According to McKinsey’s 2022 report, it has more than doubled between 2017 to 2022 (and these numbers are predicted to be higher this year).
Similarly, the computational power used to train AI has increased by a factor of 100 million in the past 10 years. According to the authors of Compute Trends Across Three Eras of Machine Learning, before 2010, the compute power we used to train AI grew in line with Moore’s Law (roughly doubling every 20 months). It is now doubling every six months. It is so powerful that it is allowing us to feed an unprecedented amount of data to LLMs in order to train them.
Through these developments, we have seen the rise of unbelievable image and video manipulation capabilities, unlike anything that we thought possible a few years ago. Interestingly, the exponential growth of AI has meant that, within the span of only a couple of years, we went from the enthusiasm and occasional apprehension of Generative Adversarial Network (GAN) image and video manipulation (who hasn’t seen a deep-fake online?) to the exciting new advancements of NLP content creation.
Large Language Models have truly pulled us deep down into the rabbit hole: generative pre-trained transformers (the GPT on the Chat-GPT) are rewriting the book on IP, productivity and – some say – creativity. Their rate of development is beyond anything anyone could have imagined.
ChatGPT-3.5 was launched in March 2022, primarily as a text-based tool. It passed the bar exams at the bottom 10th percentile. A year later, ChatGPT-4 was able to understand images and passed the bar at the top 10th percentile. Microsoft is using the power of these models to launch tools such as github Copilot for developers and, in the near future, 365 Copilot for the general public. It is likely we will use these tools to boost our productivity of everyday tasks.
These models have the ability to describe images, understand context and make suggestions based on said context, bringing forth the rise of diffusion models. The underlying advances that drove the rise of LLMs were also pushing multi-modal models with abilities and qualities that had never been seen before, resulting in the widespread use of applications such as MidJourney and Stability AI’s DreamStudio.
These models are using natural language prompts to produce incredibly intricate images and, in our case, architectural images in any style we so choose. The quality of these images is so good that there are anecdotes of small offices winning entire competitions through images created via diffusion models, and then struggling to deliver on the promise that the AI system has imagined.
In fact, there is an entirely new concept around AI and NLP called “prompt engineering”. This describes the ability to craft your prompt in such a way that it instructs the system to produce images which directly match the prompter’s expectations.
It is effectively the “art” of crafting sentences which describe what you have imagined and are closer to the computer’s understanding of what you want to see. It could be a trade that could in the future make artists and architects compete with language craftsmen and writers.
Uncertainties and unknowns
So, the question naturally arises: will AI replace architects and other key construction professionals? Before we answer that, there are some bigger – perhaps more existential – threats to consider.
Only a couple of months ago Sam Altman, CEO of OpenAI, talked to the US Senate and was calling for regulations around AI. This is because scientists fear that artificial general intelligence will bring about the singularity: a “hypothetical future when technological growth becomes uncontrollable and irreversible, resulting in unforeseeable changes to human civilisation”.
Every year, surveys from people in the AI field, consistently place this scenario before the year 2060. This latent threat has created a very polarised environment, with half of the community thinking that AI poses an apocalyptic risk, while the other half believing these concerns are exaggerated and disruptive. I believe that, as with everything, the truth is somewhere in between. The grey areas are, after all, so much more interesting.
There are efforts to mitigate the “existential risk” that AI poses. You may have read of AI alignment, that aims to align AI systems’ goals with human values, or even AI safety and fairness, which reviews how these systems safely and fairly respond to what we ask of them.
We need to intensify our work in these areas, as AI is not going anywhere. The stakes are simply too high. It is a race driven by the promise of posterity and money. AI start-ups were once making tens of millions; today they make tens of billions.
But, whether you are a fan of Peter Pan or Battlestar Galactica, you know that “all of this has happened before, and it will happen again”. Many have compared the revolutionary changes brought by AI to the splitting of the atom.
However, creative minds have nothing to fear from the advent of technological revolution. The truth is that, if we play our cards right, AI is not going to replace us, but rather augment our creativity and problem-solving capabilities.
The question is how to channel this technology to become a creative assistant that augments rather than replaces our creativityTo do this, we need to take a step back and really think about how AI can impact us in a positive way. The question is how to channel this technology to become a creative assistant that augments rather than replaces our creativity.
The first step is to identify all of the things that AI is good for (automation, augmentation, facilitation) and find real business cases that could positively impact our current workflows. Experimenting with diffusion models and prompt engineering is undeniably alluring, as we can produce incredibly exciting outputs with minimum effort. However, we need to understand the business case behind this and how it can enhance our current workflows.
According to my colleague Sherif Tarabishy, associate partner and the design systems analyst specialising in AI/ML for the Applied R+D group at Foster + Partners: “Deploying and monitoring machine learning models in a production environment is complex. This is why it is more beneficial to create a simple model that addresses a core business need, rather than focusing on complex, state-of-the-art models that don’t have a clear use case.
“Involving domain experts across the business early on is very important to identify and evaluate those impactful business use cases. They provide critical context and an understanding of the problem domain. They also help to identify the best data governance framework for a use-case, managing availability, usability, integrity and security of the business’ data.”
That is to say that there are many areas beyond image creation where AI and ML are expected to impact the field of construction. Generative design, surrogate models, design assist models, knowledge dissemination and even business insights are just some of the areas where AI and ML can be used in our pipelines.
At Foster + Partners, we have been developing services and tools along these lines. For example, since 2019, the Applied R+D group has been publishing research around design-assist and surrogate models. Design-assist models could become collaborators in our every-day design tasks, solving difficult problems in real-time. They could suggest options as we design, automate processes or provide answers to tasks that would otherwise be time consuming and labour intensive.
These are actual problems that AI-powered design assist tools can solve for us, by automating mundane tasks and, by extension, turbo-powering productivity and allowing more time for creative tasks to take placeFor example, imagine that we have a thermoactive, passively actuated, laminate material that deforms based on varied thermal conditions. As designers, we are interested in the start and end point of the deformation (how it may look at rest and how I would like it to look when heated) – that is what defines our design.
In order to control that, we would need to know how to control the laminate layering, a process that requires non-linear analysis and quite a lot of time. Or, we could use ML to train a system to predict the laminate layering and give real-time feedback to the designer.
This was exactly the task that we identified in 2019 with Marcin Kosicki, associate partner in the Applied R+D group, and our Autodesk collaborators Panagiotis Michalatos and Amira Abdel-Rahman. How can we use ML to predict how a passively actuated material would react to variable temperature changes?
With the help of Hydra, our bespoke in-house distributed computing and optimisation system, we ran thousands of simulations to understand how thermoactivated laminates behave under varied heat conditions. We then used that data to train a deep neural network to tell us what the laminate layering should be, given a particular deformation that we required.
These are actual problems that AI-powered design assist tools can solve for us, by automating mundane tasks and, by extension, turbo-powering productivity and allowing more time for creative tasks to take place.
There is currently a plethora of new third-party software developed to yield the power of optimisation and ML to provide design assist solutions to architects and contractors. From floorplate layout and massing exploration to drawing automation and delivery, these products are looking at how these techniques can be used to either provide quick design explorations during concept or automation of tasks and processes during delivery.
Diffusion models are also perceived as design assist models, as they can go way beyond image making and prompt engineering. Imagine creating 3D models and getting suggestions on detailed visualisations as the massing is changed, directly on your CAD viewport
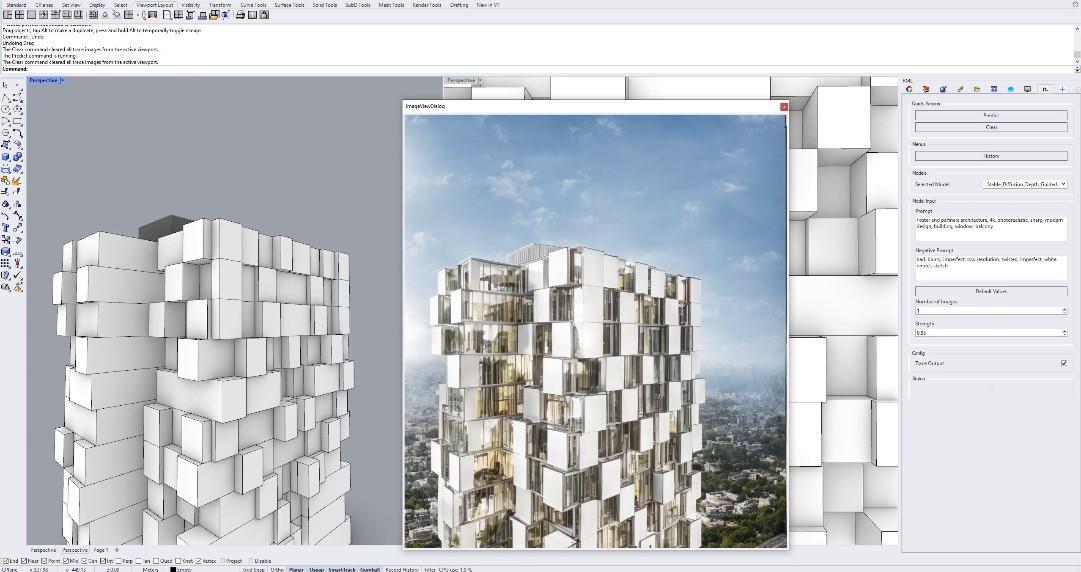
To that end, we have created a Rhino plug-in using our in-house ML inference API, which makes this possible. It allows us to quickly deploy and experiment with new ML models, with impressive results.
What is interesting about the use of diffusion models in this case is that we do not ask the computer to “imagine” a design for us. Instead, we provide the design, and we prompt for design-assist suggestions on the look and feel. The best part is that we can train the model on our own data, thereby any suggestions are inspired by our own designs and historical data.
Surrogate models can also be incredibly useful for providing real-time feedback and saving designers a lot of time. A surrogate model is an ML model which is trained to predict the outcome of what would otherwise be an analytical process.
That means that, instead of creating a model and running an analysis – for example, daylight potential – we could train a surrogate model to predict with high accuracy what the daylight potential of the massing is, without us having to export the model, run the analysis in a different software (with the interoperability challenges that this may entail) and then export the results back.
There are two components which allow us to do this: 1) high volumes of data to train the model with and 2) willingness to compromise. The former is true for any ML-trained model. The latter applies particularly to surrogate models which are meant to give a “prediction” rather than an accurate result.
If the data is rich enough and the model is properly trained, these analytical predictions can reach up to 90-95% accuracy. In any case, the user is asked to sacrifice absolute accuracy for real-time speed – a compromise most of us would be happy to make during the early design stages of a project, to make the right decisions earlier on.
We have been developing such models for analyses – like visual and spatial connectivity – since the early 2020s. With these models, we are replacing slower analytical processes with much faster and very accurate predictions. To make them accessible to everyone, we have developed a Rhino-ML plug-in that could be used by any architect at the practice.
Knowledge dissemination is another major aspect of AI. Imagine all the data – and therefore the knowledge – that an AEC practice is producing every day. A lot of that becomes untapped information saved on servers.
What if we had the chance to make all this knowledge accessible to everyone? What would it mean for a young designer to be able to ask questions that only a seasoned architect could have an answer to?
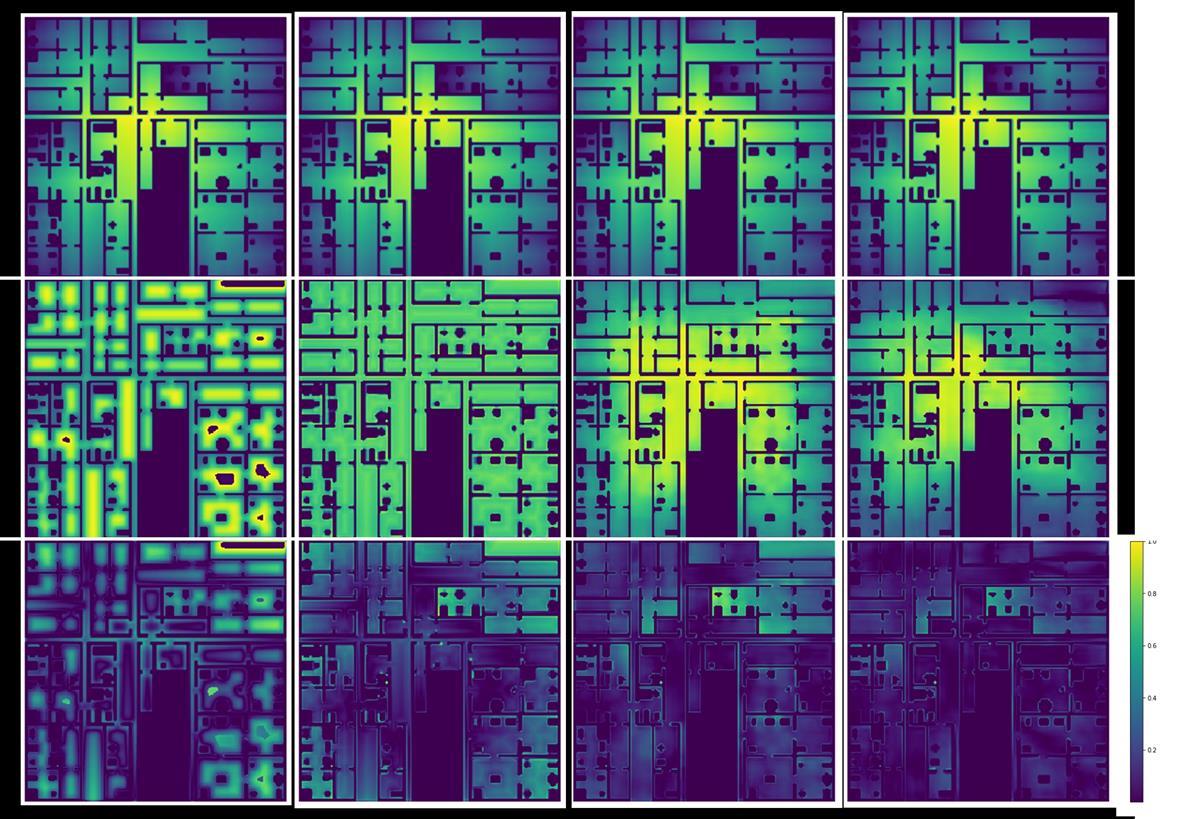
That is where the power of AI LLMs come in. With the push for LLMs and even the new focus on foundation models that have zero-shot capabilities (ie the ability to perform a set task by being presented with a couple of examples of what the user is after) now more than ever the focus is on your own data. The trick, then, is choosing the right ML model and making sure the data used is appropriately curated.
Still, this proposition should be treated with care. Some pre-trained models are prone to AI hallucinations, providing results that are not accurate, because they are trained to improvise when they do not find direct answers to questions they have been asked. This can be problematic as it means the accuracy of the response received is compromised, which can have a detrimental effect on results, depending on the context.
On the other hand, even if the model is trained not to improvise, its answers are still going to be only as good as the data it has been trained on. This puts the onus on each practice to ensure that their data has been properly curated. At Foster + Partners, we have been developing applications such as this, using different models and experimenting with how they can be deployed office wide. Many other architectural offices are following similar routes.

Finally, the other use of AI is around business insights – an application already used in every other industry. Building AI-powered applications around business data and developing predictive models to help visualise and contextualise operational data, while helping to gauge the financial aspects of a business, should be a straightforward proposition for any practice – subject to the amount of good quality data that they have.
AI is already making its mark not only on design but also during construction, operation and beyond. It can streamline the construction process by optimising schedules, predicting project costs and improving safety. But let’s face it: when we think of disruption in construction, we think of robots!
Robots in construction are not a new thing, but AI-powered robots such as those from Built Robotics, can really change the construction landscape. Additionally, anyone who has seen what Boston Dynamics’ Atlas robot (which is not actually relying on ML or AI) is capable of should have no doubt that the future efficiency of robots will be an incredible asset on-site.
The capabilities that Atlas is presenting, will allow us to fast-track production, minimise on-site risks and automate tasks in a way that augments rather than replaces people. As robots evolve, we will see that the evolution of our symbiotic relationship will depend on mutual interactions. It is the rules of engagement with them that we need to start working on – something that Asimov foresaw more than 80 years ago. However, the use of AI in construction and even operation (through the use of smart buildings and digital twins) is a wider discussion for another time.
Handling data
It is obvious that AI holds tremendous potential for transforming the way we design, construct, and experience buildings and urban spaces. But to harness AI’s power, we need to be able to control what powers it: data! Our first point of order should be understanding how to collect, organise and process our data across disciplines in a meaningful manner, so that can we leverage it.
Our datasets are growing exponentially: we produce more data than ever before during the design, construction and operation of the built environment. This is because we have also been taking advantage of the exponential growth of computational power.
By using the power of GPU computing or distributed computing – both technologies that sprung from the games and film industries – we are now capable of producing huge amounts of data, not in a matter of days or months, but in hours. This data includes thousands of solutions for projects of all scales, but also tens of thousands of analytical results that tell us how well these solutions perform.
Could we use these rich datasets to train the computer to predict optimal spatial configurations, not in a matter of hours, but within seconds? The answer is yes.
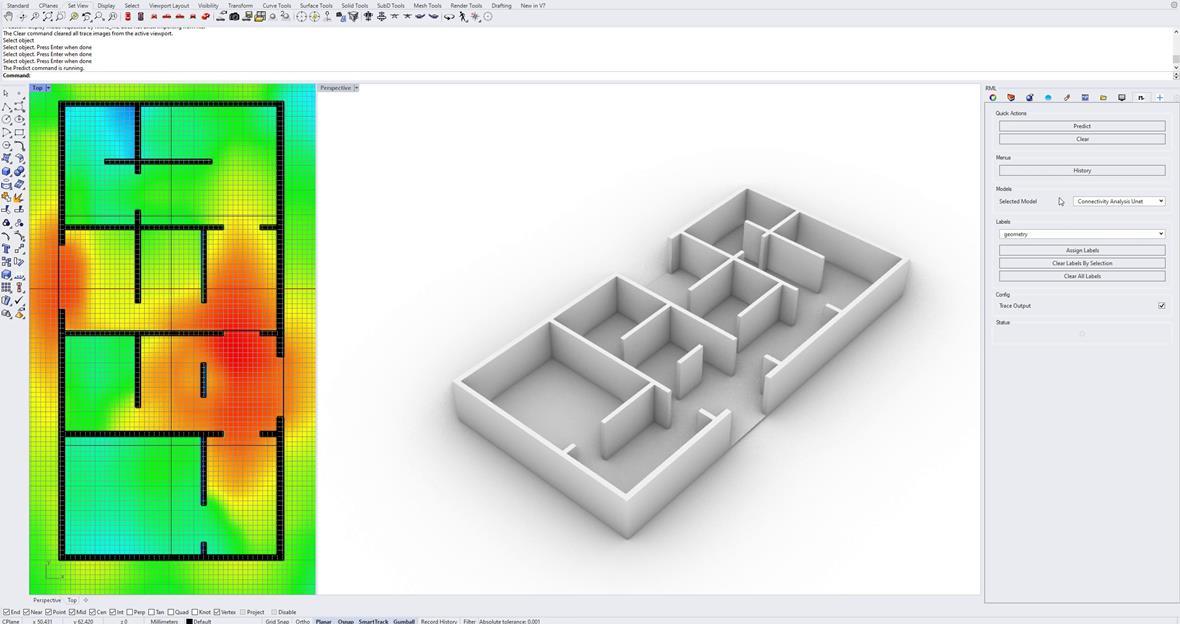
We could take advantage of the amounts of data each project yields in order to increase performance, efficiency and creativity. Many start-up companies are doing it already, by using large datasets to train their applications to provide suggestions during the design process. These AI-based generative models are going to become increasingly more prominent – and once more yield results that are only as good as the data they have been trained on.
To conclude, a more general question should probably be this: Will disruption replace creativity? This need not be the case. While these technologies can assist and enhance various aspects of the architectural process the role of the architect remains crucial, as it brings to the table not only creativity and innovation, but also aesthetics, collaboration, communication and ethics – coupled with responsibility.
To ensure that disruptive technologies augment rather than replace our creativity, we need to set up rules of engagement, similar to Asimov’s 3 laws of robotics. These will need to span data contextualisation, regulatory frameworks, IP, education, embedded biases in data and ethical considerations. There are a lot of challenges here and, in many ways, these challenges are even more crucial than the AI-based applications we are going to develop for the industry.
To begin with, data is key. How are we going to suitably train AI systems for the AECO, when the industry lacks appropriate schemas that may deliver consistent tagged building datasets that are contextualised, socially appropriate, structurally viable, sustainability sensitive and even building-code complying?
If we want to use and control these technologies to the best of our ability, we need to learn to control the data that drives them first. This is not an easy ask, but some companies are already taking up the challenge.
There is still a lot of work to be done around AI alignment, AI safety and fairness and AI ethicsThe EU has certainly started building regulatory frameworks around proper data governance. One such example is data pooling of smaller companies for AI uses, which will ensure ringfencing of their IP rights while allowing them to be competitive against bigger players.
However, there is still a lot of work to be done around AI alignment, AI safety and fairness and AI ethics, particularly in relation to embedded unconscious biases in the data we use to train these systems. At practice level, data fidelity is even more important to ensure the quality and consistency of the outcome, whether one is deploying in-house trained ML models or fine-tuning pre-trained ones.
Following on from that, IP is going to be another interesting challenge. Currently, artists all over the world are taking part in class action lawsuits against AI-based software providers contesting the use of their own proprietary data to train systems, which have become a direct competitor to their own livelihoods.
For over three months, more than 11,000 members of the Writers Guild of America have been on strike, asking for assurances that AI will not take their roles as scriptwriters. And even as I write, actors from the Screen Actors Guild - American Federation of Television and Radio Artists are on strike, contesting the use of AI to create actors’ likenesses virtually and without any humans involved. To have both guilds (writers and actors) on strike simultaneously has not happened for 60 years.
These fuzzy IP boundaries when it comes to AI are derived from how data is used and the lack of any kind of robust legal or regulatory frameworks around the use of these technologies in any industry. And, to top that, there are also several ethical considerations that have already surfaced through the use of AI, mainly revolving around reinforcement of bias and discrimination. There are many such documented cases spanning from recruitment to criminal justice, and we can only be certain that our industry’s data will have embedded biases too.
These are big questions and require decisive action at policy level. Hopefully, we can push these discussions sooner rather than later, as the time for pre-emptive action is already behind us.
Finally, we cannot shy away from the role that education has to play in the way these new technologies are adopted and implemented by the industry. There are already courses which focus on how AI applications could be used for architectural purposes.
This, in and of itself, is not a bad thing: we should be embracing disruptive tech and trying to understand how it will impact our industry. But the educational framework for this to happen properly is not there yet – simply by virtue of how fast this discussion is moving. The bottom line is that, if educators’ objective is augmentation rather than replacement of creativity (which is in many cases the by-product of the fascination these technologies hold), we are yet to write ourselves outside of the equation.
The answer to how AI is going to affect our profession is going to depend on us and how actively we try to channel this incredible technological advancement for the improvement of the AECO. We do live in exciting times, and we need to seize this moment by driving change – rather than being swept away by it. To do that, we must be active participants in bringing forward the change we want to see.
We are already riding a wave of unprecedented exponential acceleration – the stakes are too high, but so can be the rewards.
Source: Webvision Cloud
Original Content:
https://shorturl.at/nrtMZ