Hillary
Posted on July 21, 2023
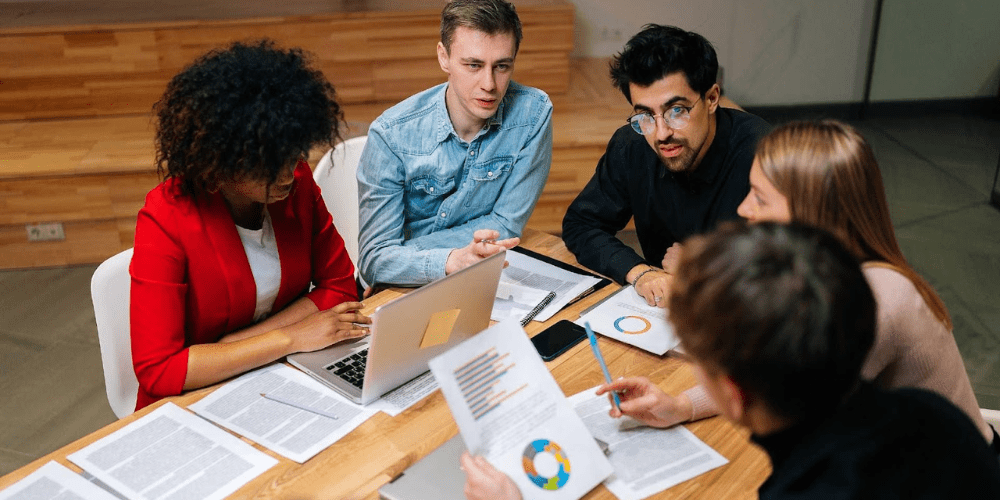
Are you tired of pouring over mountains of blog data without any meaningful insights to show for it? Do you wish there was a way to turn that raw data into actionable strategies and measurable results? Look no further! In this blog post, we will share with you some valuable tips and tricks for effective blog data analysis. From demystifying the complexities of raw data interpretation to unveiling hidden patterns and trends, get ready to transform your blogging game like never before.
Introduction to Blog Data AnalysisAssuming you have access to your blog’s raw data, the first step is to decide which metric(s) you want to track. For example, you might want to track pageviews, unique visitors, or time on site. Once you’ve decided which metric(s) to track, you can begin using a data analysis tool like Google Analytics to generate reports.
The next step is to actually interpret the data in your reports. What do the numbers mean? Are they good or bad? To help you answer these questions, it’s helpful to set benchmarks. For example, if you’re tracking unique visitors, you might want to compare your current month’s worth of data to the same month from the previous year. This will give you a sense of whether your traffic is growing or shrinking.
Once you’ve interpreted your data and set benchmarks, it’s time to take action! Use what you’ve learned from your analysis to make decisions about how to improve your blog. For example, if you see that traffic has decreased since last month, maybe it’s time to start promoting your blog more aggressively on social media.
Setting up and Identifying the Goals of Data AnalysisSetting up and identifying the goals of data analysis can seem like a daunting task, but it doesn’t have to be. By following a few simple steps and having a clear understanding of what you want to achieve, you can easily set up a data analysis plan that will help you get the most out of your data.
The first step is to identify the goals of your data analysis. What do you want to learn from your data? What questions do you hope to answer? Once you have a clear understanding of your goals, you can begin to develop a plan for how to best collect and analyze your data.
Next, you need to choose the right tools for collecting and analyzing your data. There are many different software programs and online services that can help you with this task. Do some research and select the tools that will work best for your needs.
Once you have all of the necessary tools in place, it’s time to start collecting and analyzing your data. You should create a clear structure and timeline for how you will collect and analyze the data, as this will prevent you from getting overwhelmed by the sheer amount of information that needs to be processed.
Once you have collected and analyzed your data, it’s time to draw conclusions and make decisions based on your findings. Keep in mind that the data analysis process is an iterative one, so be prepared to adjust your plan as needed based on what you learn from the results of each stage of your analysis.
By following these steps, you can easily set up a data analysis plan that will help you get the most out of your data. All it takes is some planning and preparation before diving into the deep end.
Types of Data Analysis ToolsThere are many different types of data analysis tools available to blog owners and content creators. The most important thing is to choose the right tool for the job at hand. Here are some of the most popular data analysis tools used by bloggers:
-Raw data: This is the most basic type of data analysis tool. It simply allows you to see all of the data that has been collected about your blog in one place. This can be useful for getting a general idea of how your blog is performing, but it can be overwhelming and difficult to interpret.
-Analytics software: This type of tool helps you to track and analyze specific metrics about your blog. This can be anything from pageviews and unique visitors, to bounce rate and time on site. analytics software can be very helpful for understanding which areas of your blog are performing well and where there is room for improvement.
-A/B testing: This is a technique that allows you to test two different versions of a piece of content or design on your blog to see which performs better. A/B testing can be very helpful for fine-tuning your blog to get the best results possible.
– Heatmaps: A heatmap is a visual representation of where people are clicking on your website or landing page. This can be extremely helpful for understanding what parts of your site are most popular and which ones need more attention.
-Social media analytics: Many tools are available specifically for tracking your social media performance. These tools can be very helpful for understanding which of your social media posts are the most popular, and which of your followers are the most engaged.
Tips for Cleaning and Analyzing DataData analysis can be a daunting task, especially if you’re not sure where to start. This blog post will give you some tips and tricks for cleaning and analyzing data, so you can get the most out of your data.
1. Start with a clean dataset. This may seem obvious, but it’s important to make sure your data is clean before you start doing any analysis. If your data is full of errors, it will be very difficult to get accurate results. So take some time to clean up your data before you start analyzing it.
2. Know what you want to analyze. Before you start looking at your data, think about what you want to achieve with your analysis. What are your goals? What questions do you want to answer? Once you know what you want to accomplish, it will be easier to choose the right tools and methods for your analysis.
3. Choose the right tools for the job. There are many different ways to analyze data, and there is no one-size-fits-all solution. Choose the tools that are best suited for the task at hand. If you’re not sure which tools to use, there are many resources available online that can help you choose the right ones for your needs.
4. Be prepared to iterate on your analysis. It’s rare that everything goes according to plan when performing data analysis. You may need to try several different approaches before finding one that works well for your dataset and goals . Don’t be afraid to experiment and iterate on your approach until you find one that gives you the results that you want.
5. Keep your data organized. If you have a large dataset, it can be easy to get lost in the details. Make sure to keep your data organized so that you can easily find what you’re looking for when needed. You should also make sure to document any changes or updates to the data so that you can refer back to them later if needed.
Following these tips will help ensure that your data analysis is successful and provides the answers you need. However, don’t be afraid to try new methods if they seem promising. Data analysis is a continuous process and requires trial and error at times, but with practice, you will quickly become an expert.
Blog Analytics ReportingBlog analytics reporting can be a powerful tool for bloggers who want to track their progress and understand what is working well on their blog. Data analysis can help identify patterns and trends in your audience’s behavior, such as what content is most popular, what time of day they are most active, and where they are coming from.
To get started with data analysis, first you need to set up tracking on your blog. Google Analytics is a free and popular option. Once you have Analytics set up, you can start exploring your data. The default dashboard gives you an overview of your site’s traffic, including pageviews, unique visitors, and average time on site. You can also see how your traffic has changed over time.
Drilling down into your data can help you understand which of your blog posts are performing the best. To do this, go to the Behavior > Site Content > All Pages report in Google Analytics. This report shows you pageviews and other engagement metrics for each of your blog posts. You can use this information to identify which topics are resonating with your audience and adapt your content strategy accordingly.
In addition to looking at individual blog posts, you can also use analytics to understand broader trends on your site. For example, the Acquisition > Overview report shows you where your traffic is coming from (e.g., search engines, social media, direct). This information can help you optimize your marketing efforts and better understand which channels are driving the most traffic to your site.
Finally, analytics reporting can also help you understand the demographics of your audience. The Audience > Demographics report in Analytics shows you data about visitors including gender, age group, and location. This information can give you a better idea of who is visiting your blog and how they are engaging with it.
Implementing Changes Based on Insights Obtained From Data Analysis ReportsData analysis can be a complex and time-consuming process, but it is essential for understanding how your blog is performing and what changes you can make to improve it. By understanding the data contained in your reports, you can identify areas where your blog could be improved and make changes accordingly.
There are a few things to keep in mind when implementing changes based on data insights:
1. Be sure to understand the data before making any changes. Insights from data can be helpful, but they won’t mean anything if you don’t understand what they’re trying to tell you. If you’re not sure about something, don’t hesitate to ask someone who knows more about data analysis.
2. Keep your target audience in mind when making changes. The goal of your blog is to serve your target audience, so be sure that any changes you make will actually benefit them. If you’re not sure whether a change will be beneficial, try running a test on a small group of users before rolling it out to everyone.
3. Make sure you have a plan for how you’ll implement the changes. Once you’ve identified areas for improvement, come up with a plan for how you’ll address them. This plan should include specific steps that need to be taken and timelines for each step. Trying to make too many changes at once can be overwhelming and lead to mistakes, so it’s important to take things one step at a time.
4. Be prepared to adjust your strategy as needed. If you find that the changes you’ve implemented aren’t having the desired results, don’t hesitate to adjust your strategy. This could mean tweaking the changes or even reversing course altogether. Data can be a powerful tool, but it’s important to remember that what works in one situation may not work for another.
By being mindful of these tips, you’ll be able to use data insights to make meaningful improvements to your blog and ensure that your content is targeted at the right audience.
ConclusionBlog data analysis can be a powerful tool for unlocking insights that help you further optimize your blog. Taking the time to organize and analyze data from multiple sources allows you to gain deeper insight into the performance of your blog, giving you an edge over competitors who aren’t taking the same approach. With a few simple tips and tricks under your belt, anyone can transform raw data into actionable insights in no time. Start putting these tactics to work on your blog today—you’ll wonder how you ever made it this far without them.
Source: TechBullion
Original Content: https://shorturl.at/awOV9